Don't let generative AI limit your team's creativity
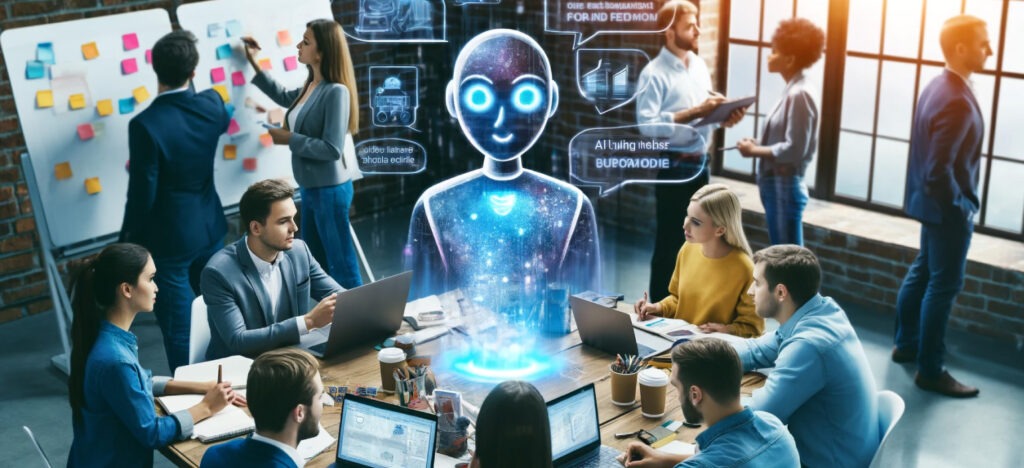
No one doubts ChatGPT's ability to generate a lot of ideas. But are those ideas any good? In a recent real-world experiment, teams participating in a creative problem-solving task saw modest benefits from AI assistance for the most part, and some even underperformed.
Don't blame the technology, says Kian Gohar, CEO of leadership development firm GeoLab and one of the study's authors. Common misconceptions about generative AI, problem solving and the creative process are causing workers and their managers to use the tools incorrectly, sometimes leaving them worse off than if they had proceeded without the help of AI.
Gohar and his co-researcher, Jeremy Utley of Stanford University, partnered with four companies: two in Europe and two in the United States. Up to 60 employees at each company were invited to work in small teams on a business problem facing their company: how to develop internal training resources, for example, or how to increase B2B sales of a particular product. In each of the companies, some of the teams (those in the control group) tackled the problem without any AI assistance, while others (those in the experimental group) used an open source version of ChatGPT. All teams saw a brief presentation on the problem they were to address and had information sheets with relevant details.
Teams had 90 minutes to generate potential solutions, following a structure prescribed by the researchers. Employees first worked individually and then shared their ideas with their peers during a brainstorming session. Teams in the experimental groups were able to use ChatGPT during both ideation phases, and were encouraged to train the tool on the problem by entering material from the information sheets. At the end of the exercise, each team presented their ideas.
The "owner" of each problem-the person in each organization responsible for implementing the eventual solution-judged the ideas, assigning grades from A ("highly compelling") to D ("not worth pursuing") without knowing which ones had emerged from human-machine collaborations. The results defied the researchers' expectations, Gohar says. He and his colleagues had assumed that teams leveraging ChatGPT would generate many more and better ideas than others. But those teams produced, on average, only 8% more ideas than teams in the control group. They got 7% less of D, but they also got 8% more of B ("interesting but needs development") and about the same proportion of C ("needs significant development"). Most surprisingly, they got 2% less of A. "Generative AI helped workers avoid terrible ideas, but it also led to more average ideas," says Gohar. Surveys conducted before and after the exercise showed that teams that used AI gained much more confidence in their problem-solving abilities than others-a 21% difference. But the ratings they received suggest that much of that confidence was misplaced.
"Brainstorming with generative AI requires rethinking your ideation workflow and learning new skills," Gohar concludes. "But if you approach it as a structured, ongoing conversation, you can access an amazing ability to develop better, more creative ideas faster."
Of course, the potential for generative AI in problem solving is real, says Gohar. Here are some steps to maximize it.
Be precise about the problem you want to solve. The large language models that underpin generative AI chatbots are designed to give "average" responses; their algorithms have been trained to identify the highest probability of sequential words. If one types, "I bark like a..." and asks the bot to complete the thought, it will almost certainly offer the word "dog." But if teams are looking for innovative solutions, the average answers will be of little use.
Therefore, managers should teach their teams to formulate very specific problem statements, including as many details as possible, before interacting with the tool. For example, instead of asking, "How can we improve customer satisfaction?" teams could say, "Our customer journey includes the following steps... What changes in our onboarding step will improve retention in a 10%?" Gohar comments, "People expect AI to be an oracle: plug it in, and it will give you your solution." Teams that took that approach-simply stating the problem in general terms and asking ChatGPT to solve it-obtained mediocre results.
Spend time brainstorming individually without the bot. Before interacting with the AI, give team members time-from 15 minutes to half an hour, say-to individually come up with ideas. That will help ensure that they approach the team meeting and AI deployment without being affected by groupthink or what the tool suggests. This step is crucial for gathering diverse and creative ideas and maximizes the number of unique ideas that are brought to the group for discussion.
Rigorously train AI. Generative AI systems lack the contextual understanding that people acquire over months or years of working in their organizations and industries. Before integrating ChatGPT or a similar tool into the ideation process, you need to help it get up to speed. Enter as much data related to your specific problem as you can. That might include the thinking of a group of customers, past successes and failed initiatives, and industry benchmarks.
Approach AI as an ongoing conversation partner, not an oracle. Teams in the study developed better ideas when they interacted multiple times with ChatGPT. "Most problem solving requires a conversation," Gohar says. "You'd have a discussion with your colleagues to come up with a better solution to a problem, and that holds true when one of those colleagues is ChatGPT."
Many of the teams in the experiment simply accepted the first suggestion ChatGPT offered. Gohar attributes this to the Einstellung effect: a cognitive bias whereby people gravitate toward early, familiar solutions rather than exploring possibilities more expansively. That probably contributed to the high rate of B-grade ideas generated by AI-assisted teams. No matter how good the tool's initial suggestion seems, teams should always follow up with more and more specific questions, Gohar says. Doing so allows the model to refine its answers and gives users more solutions to ultimately choose from. "The teams that got A's were those that had interactive conversations with the bot," Gohar emphasizes.
Have someone from outside the team facilitate the final decision. When the team meets to share possible solutions, designate a member to consolidate suggestions. Then ask the AI to analyze them for alignment with your objectives, offer critiques, challenge assumptions and suggest more alternatives. This step also serves as a training mechanism and will improve the model's future performance. It can be helpful, Gohar says, to have an external facilitator-someone who doesn't have a vested interest in the outcome, who ideally is well versed in AI ideation-to guide the process, help prioritize ideas and plan next steps.
Collaboration: Grupo Auge | HBR.